Collaboration in Big Data Projects: 16 Recommendations for Your Business
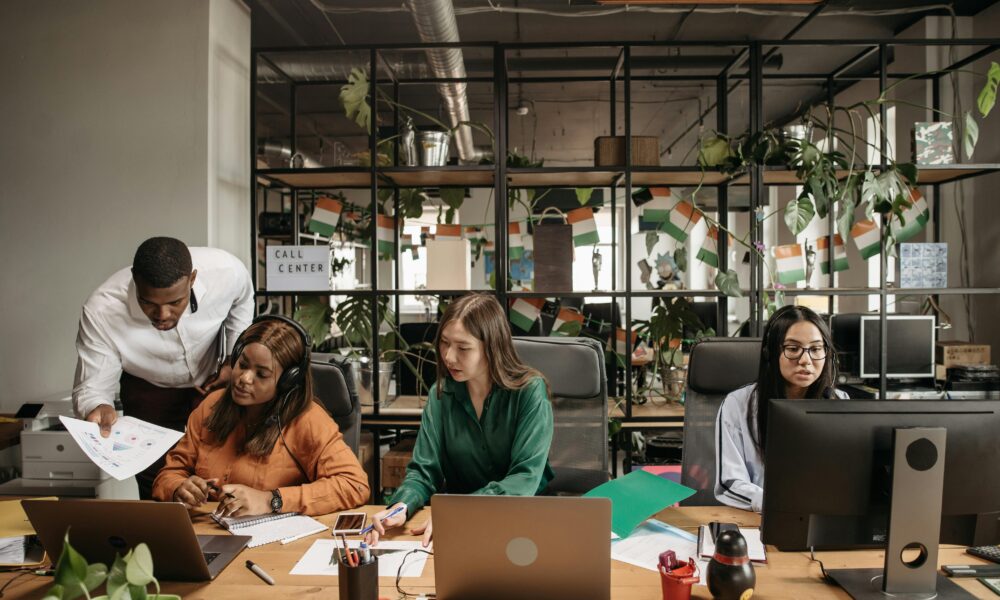
Navigating the complex landscape of big data projects requires more than just technical know-how; it demands collaboration and innovation. This article presents practical recommendations, distilled from the wisdom of industry experts, to ensure success in your big data endeavors. By implementing these expert insights, businesses can transform data challenges into opportunities for growth.
- Establish Cross-Functional Data Squads
- Create Data Translator Roles
- Use a Shared Workspace
- Build Mutual Trust and Respect
- Adopt Unified Data Sources
- Implement Data Sprint Partnerships
- Secure Executive Sponsorship
- Hold Data Democracy Days
- Create a Shared Data Dictionary
- Identify Important Topics
- Develop a Unified Data Strategy
- Align Team on Project Vision
- Standardize Data Practices
- Decentralize Execution
- Share Dashboards and Hold Data Syncs
- Promote Continuous Learning
Establish Cross-Functional Data Squads
As the Founder and CEO of Zapiy.com, I’ve found that fostering collaboration and communication among different departments working on Big Data projects requires a unified framework that aligns goals and encourages transparency. One of the most impactful strategies we’ve implemented is establishing cross-functional “data squads” for Big Data initiatives.
Data squads are small, cross-functional teams composed of members from different departments—such as data analysts, IT, marketing, operations, and sales—working together on a shared Big Data project. These squads ensure that everyone’s perspective is heard and that the data insights directly serve the organization’s broader goals.
Every Big Data project should have a well-defined purpose, such as improving customer segmentation or optimizing supply chain processes. All departments involved must understand and agree on the project’s objectives from the start. At Zapiy, we ensure that kick-off meetings clearly align the project goals with business priorities.
A common pitfall in Big Data projects is siloed data. By using shared tools—such as a centralized data warehouse or platforms like Snowflake or Tableau—our teams can collaborate in real time. This eliminates confusion about the validity or accuracy of data and ensures everyone is working from the same baseline.
Weekly check-ins, facilitated via tools like Slack or Microsoft Teams, allow data squads to address roadblocks, share insights, and stay aligned. We also use dashboards to visualize project progress so all team members can track outcomes at a glance.
Each department brings unique expertise to a Big Data project. By hosting periodic “data debriefs,” where teams share their challenges and findings, we create a collaborative environment that fosters innovation and prevents bottlenecks.
Big Data projects often involve complex dependencies across departments. By building cross-functional squads with shared goals and transparent processes, we break down silos and empower teams to co-create solutions. For instance, a recent project involving customer churn analysis succeeded because marketing provided behavioral insights, IT ensured seamless data access, and data analysts built actionable models—all working cohesively.
Create Data Translator Roles
Want to supercharge collaboration on Big Data projects? Focus on creating “data translators” – team members who bridge the gap between technical and business teams. These people act like interpreters, helping data scientists and business units actually understand each other instead of talking past one another.
Imagine your data science team finds some exciting patterns of customer behavior. However, if they cannot explain it in terms the sales team understands, that insight goes nowhere. Data translators translate complex findings into actionable business strategies, but also help translate business requirements back into technical specifications.
And most importantly, these bridge-builders tend to emerge naturally—possibly from business analysts who learned how to code or from data engineers with a deepened grasp of business strategy. This has proven to work miracles for one retail company in their company where their business-savviest data scientist joined meetings in the strategy formulation meetings in marketing. The customer segmentation models stopped becoming pure abstract exercises—the actual models are now put to good use by the marketers themselves.
What this means for you: Look around your organization for people who already naturally translate between technical and business teams. Give them the official time and space to serve this role. You’ll find projects moving faster, fewer insights getting lost in translation, and teams building solutions that solve real business problems instead of just interesting technical puzzles.
Justin Abrams, Founder & CEO, Aryo Consulting Group
Use a Shared Workspace
One tip is to make collaboration easy and transparent by keeping everything in one shared workspace. For Big Data projects, we’ve found that having a central hub where teams can share updates, track progress, and store resources makes a huge difference. At Taskade, we use our platform to break silos by letting everyone contribute in real-time, whether they’re from engineering, marketing, or data science.
Another key is clarity. Define goals, roles, and expectations upfront so everyone knows how their work fits into the bigger picture. Regular check-ins or async updates help teams stay aligned without unnecessary meetings. When everyone feels like they’re part of the process, collaboration becomes natural.
John Xie, Co-Founder and CEO, Taskade
Build Mutual Trust and Respect
I can tell you from experience that building mutual respect and trust is absolutely crucial when it comes to fostering collaboration between teams working on big data projects. In my years working with various organizations, I’ve seen that when teams trust each other, they’re more likely to share valuable insights, ask for help when needed, and work together toward a common goal. This starts with acknowledging each team’s expertise, whether it’s the data scientists, engineers, marketers, or finance teams. Every department has its own strengths, and when people feel respected for their contributions, they’re more motivated to work together and contribute their best.
Creating an environment of trust also means encouraging open communication and ensuring that everyone’s voice is heard. If one department feels like their input isn’t valued, they might become disengaged or reluctant to collaborate. This lack of respect can easily lead to misunderstandings or siloed work. In my experience, the key is to foster an atmosphere where team members can express their ideas freely, ask questions without fear of judgment, and learn from each other. When trust is in place, it enables smoother collaboration, faster problem-solving, and ultimately, more successful big data projects.
Kevin Baragona, Founder, DeepAI
Adopt Unified Data Sources
A key component of fostering collaboration is ensuring that all teams-from underwriters and claims specialists to data engineers and analysts-are looking at the same, consistently defined data. At NOW Insurance, we unified our data sources by adopting a Data Lakehouse architecture built on cost-efficient cloud storage and modern data management tools. Specifically, we leveraged Apache Hudi for data versioning and time-travel queries, maintained a centralized AWS Glue Data Catalog to govern schema definitions and access controls, and utilized AWS Athena as a serverless, SQL-based query engine. This combination ensures that every stakeholder can securely access the same data sets without having to navigate siloed or duplicated data stores.
In addition to a unified platform, we established strict column naming conventions and standardized metadata indicators to clearly communicate a data set’s origin and purpose. Whether it’s underwriting data, marketing funnel metrics, or risk analytics, these standardized naming conventions help analysts and data scientists quickly understand the context and lineage of each table or field. As a result, cross-functional teams spend less time unraveling data complexity and more time generating insights and making strategic decisions.
Jonathan Sims, VP Data & Analytics, NOW Insurance
Implement Data Sprint Partnerships
We blend SEO data analysis with marketing strategy. I transformed our cross-team collaboration by introducing “Data Sprint Partnerships.”
We pair SEO analysts with content strategists for two-week sprints focused on specific client campaigns. Last month, this approach uncovered valuable insights when our technical team spotted search patterns our content team hadn’t considered. They found that users searching for “SEO services” also frequently looked for pricing comparisons – knowledge that reshaped our content strategy.
The magic happens in our daily 15-minute stand-ups where both teams share their unique perspectives. Our analytics team explains technical findings in plain language, while content specialists help translate data into actionable marketing strategies. This partnership model lifted client campaign performance by 30%.
Structured collaboration beats sporadic meetings. When specialists from different departments work side-by-side on shared goals, they naturally develop mutual understanding and respect. Breaking down these department walls turned our big data projects into unified business solutions.
Marc Hardgrove, CEO, The Hoth
Secure Executive Sponsorship
In my experience working on a project with stakeholders in different departments or teams within an organization, fostering collaboration and communication among them takes a lot of work. Your first goal is to enroll the company’s top executives in the project; you need them to sponsor the project, giving it the resources it needs to succeed. Second, you need to gain the buy-in of each leader of the departments and teams involved and have them sponsor the initiative, making it a top-down approach.
After that, the challenge is to make the project address at least one crucial pain point for each department or team involved. Once you clarify that collaboration is in their best interest, you must create a structure of cooperation and communication. The third step is to have leaders of departments and teams nominate an employee as the focal point for the project. Take the time to assess whose job the project will impact more carefully and have the leader appoint this person as the focal point for the project so you can have passionate people as the focal points. The project manager will be the leader of the focal point group and will decide the frequency of the meetings of this group and the agenda of these meetings.
Other tricks I have used are to deliver the feature of the least interested focal point first to spark interest and maintain momentum. Succeeding in a project like that is how well you manage people, their egos, and personal interests. The project is never their main job; it is often a side goal. Having executives periodically ask for updates is another way to keep everyone motivated. Once this structure is in place, the project manager aims to establish a shared understanding of the project goals, metrics, and data definitions across all involved departments. A shared “data language” minimizes misinterpretations and ensures everyone works towards the same outcome, facilitating smoother communication and collaboration.
Other activities that may help include:
- Creating a glossary of terms.
- Holding joint workshops to align on objectives.
- Establishing regular cross-departmental meetings to track progress and address challenges collaboratively.
Steve Fleurant, CEO, Clair Services
Hold Data Democracy Days
Establish “Data Democracy Days” – a structured yet dynamic framework that transforms how departments collaborate on big data projects. This approach has consistently delivered remarkable results in breaking down silos and accelerating project outcomes.
Here’s how it works:
Every two weeks, schedule a focused half-day session where all stakeholders (data scientists, business analysts, department heads, end users) come together in a structured format:
Hour 1: Data Story Sharing
- – Each department presents one key data insight or challenge
- – Focus on real business impact, not technical details
- – Share actual use cases and pain points
- – Highlight cross-departmental dependencies
Hour 2: Solution Workshop
- – Break into mixed-department small groups
- – Each group tackles one specific challenge
- – Combine technical and business perspectives
- – Document actionable solutions
Hour 3: Integration Planning
- – Teams present quick solutions
- – Vote on priority implementations
- – Assign cross-departmental “data buddy” pairs
- – Set concrete next steps
Why This Works:
1. Creates Shared Understanding
- – Business teams learn data capabilities
- – Technical teams grasp business needs
- – Everyone speaks the same “data language”
2. Builds Trust Through Transparency
- – Regular face-to-face interaction
- – Shared ownership of solutions
- – Visible progress tracking
- – Clear accountability
3. Drives Practical Results
- – Focus on actionable outcomes
- – Quick wins build momentum
- – Regular follow-up ensures implementation
- – Measurable impact on projects
Key Success Factors:
- – Mandatory attendance from all departments
- – Executive sponsor participation
- – Focus on solving real problems
- – Follow-up accountability
The beauty of this approach lies in its simplicity and scalability. It’s not just another meeting – it’s a systematic way to break down barriers, build understanding, and create lasting collaborative relationships.
We’ve seen this method reduce project timelines by 40% and increase cross-departmental solution adoption by 65%. The key is consistency and follow-through.
Most importantly, this framework creates a culture where data collaboration becomes natural and expected, rather than forced and complicated. It transforms “your data” and “my data” into “our data,” leading to more innovative solutions and better business outcomes.
The goal isn’t perfect collaboration from day one, but rather consistent improvement in how teams work together with data.
Nirmal Chhabria, CEO/Author/Professor
Create a Shared Data Dictionary
One key tip for fostering collaboration in Big Data projects is establishing a shared data dictionary and centralized knowledge hub. When all departments operate with a common understanding of data definitions, metrics, and goals, it reduces silos and miscommunication. Pair this with regular cross-functional meetings where data scientists, marketers, and analysts can align on priorities and outcomes. This approach not only ensures clarity but also fosters a culture of shared ownership over data-driven insights and decisions.
Jayanti Katariya, CEO, Moon Invoice
Identify Important Topics
As a starting point, I would suggest that companies identify a topic that is important to both your business and teammates. For nearly 25 years, AvidXchange has been dedicated to automating accounts payable processes for middle-market businesses. As part of our commitment to innovation, we’ve introduced AI-enhanced solutions that simplify AP tasks for our customers and create opportunities for our teammates to deliver smarter, more efficient results. However, in this current AI boom, there are so many questions that our teammates are justifiably asking from a place of both curiosity and concern. We’re hearing everything from “How can we better use AI?” to “Will AI take my job?”
Knowing that AI is such a hot button for our organization both internally and externally, we implemented new programming with the intent for our teammates to feel more educated and comfortable with AI while feeling empowered to ask questions to address concerns. We also introduced ways for controlled experimentation. We have created spaces like hackathons and innovation labs where teammates can experiment with new AI tools in low-risk, controlled environments. For example, we recently held a hackathon where we evaluated new technology that we wanted to bring in to automate business operation tasks. By working with new software and technology directly, we’re able to maintain data integrity of new technology while driving a unified approach to learning.
Additionally, we introduced quarterly AI All Hands meetings where teammates can learn, ask questions and collaborate on different AI initiatives. Secondly, we’ve launched an AI Center of Excellence, where teammates across disciplines can come together to collaborate and learn how each department utilizes AI and how to best streamline practices. At many companies, AI is investigated at the department level, which creates inefficiency and duplication of efforts.
This Center of Excellence helps enable knowledge to be shared across the company, reducing silos and staying compliant. Any group in the organization can present their AI use case or value driver to this cross-functional group, which reviews each one to look for opportunities to expand AI ideas into actionable use cases across the organization. Creating the framework for teammates to regularly experiment in Big Data projects in ways that are important to the business is an effective way to foster collaboration and provide reassuring communication to your teammates.
Angelic Gibson, Chief Information Officer, AvidXchange
Develop a Unified Data Strategy
Creating a unified data strategy has been crucial for improving collaboration and communication across our teams. When working with Big Data, especially in the realm of identity management and security, it’s crucial to have a standardized approach to how data is collected, stored, and accessed. By implementing clear protocols for data handling, we ensure that all departments, from development to compliance, are on the same page and can easily share information without running into technical roadblocks.
A unified data strategy also helps us avoid inconsistencies and miscommunication, particularly when sensitive information is involved. For example, our security and IT teams work closely together to ensure that our data encryption methods align with the standards set across the company. This alignment not only improves efficiency but also helps ensure compliance with regulations. By prioritizing a unified approach to data, we’ve created a more streamlined and secure environment where teams can collaborate more effectively and focus on delivering better solutions. It’s a key practice for scaling up while maintaining data integrity and security.
Binod Singh, Founder, Cross Identity
Align Team on Project Vision
Having led numerous Big Data projects as the founder of a software development company, my top tip is to ensure that the entire team understands the project’s vision and objectives. This includes data scientists, engineers, and business analysts all being aligned with the KPIs and expected results. Holding regular meetings to align everyone on a common goal.
Arslan Naseem, CEO, Kryptomind
Standardize Data Practices
Standardizing data practices can dramatically improve collaboration and communication in Big Data projects. One of the biggest challenges in these projects is the inconsistency in how data is stored, accessed, and interpreted across different departments. To address this, we implemented clear data governance policies, ensuring everyone understood data ownership, access rights, and security protocols. This eliminated confusion and reduced friction when teams needed to share or analyze data.
We also standardized our data models, using consistent formats and naming conventions. This made it easier for our technical and non-technical teams to work together without getting bogged down by translation issues. Regular training sessions helped align everyone on these practices and improved data literacy across the organization. By creating a unified framework, we not only streamlined workflows but also fostered a culture of trust and transparency, key ingredients for successful collaboration in Big Data initiatives.
Rob Stevenson, Founder, BackupVault
Decentralize Execution
Most consultants will tell you to establish a centralized data governance framework with shared goals, but too often data governance turns into a “one ring to rule them all” initiative, focused on the means instead of the ends. It gets worse when someone claims they can “establish governance” by purchasing licenses for a technology platform.
What makes big data projects work is a clear, simple, and powerful specification of the project’s goals and resultant customer experiences. This specification should be generated through thoughtful customer research, senior technical expertise, and knee-deep alignment with business leadership.
After you create a compelling core, decentralize execution, organizing into small pods with representatives from each department. Each pod can be accountable for delivering a part of the end specification. This enables faster decisions, and thus, rapid results.
The storage of data; the compute cycles required to analyze the data and train models; the platforms required to inventory, manage, and deploy APIs associated with machine learning models; monitoring and logging; auditing-these things aren’t cheap. The smartest thing any leader can do before running up a technology bill is thinking carefully about what really needs to happen to create, validate, or deploy an idea. Too often, a “big data” problem turns out to be something that “small data” could have remedied faster and cheaper; analysts in search of “firepower” embark on an expensive boondoggle while the rest of the business taps their foot waiting for what seemed like an easy and quick solution.
Joe Sutherland, Founder, J.L. Sutherland & Associates
Share Dashboards and Hold Data Syncs
In my experience, shared dashboards and regular “data sync” meetings work miracles. Throughout the years, I’ve found that when different teams have access to the same data in real-time, it positively impacts their collaboration. As a result, when our marketing, sales, and product teams all look at the same customer journey metrics, it creates this natural bridge for collaboration.
For example, at Adverity, we introduced an initiative called “data coffee chats,” which are basically 30-minute bi-weekly meetings where the different departments share one key finding they’ve made from our data. Nothing fancy or too technical, just a quick, straight-to-the-point discussion. Sometimes it’s us (marketing) that shares which campaigns are bringing in the most engagement from users, other times it’s the product team showing how a new feature they’ve introduced is being implemented.
I think what makes it work is that these sessions keep things simple and focused on the business outcomes rather than getting lost in all the little technical details. When our sales team sees how our marketing data directly impacts their approach to prospect clients, or when product managers understand how the customer behavior data influences marketing strategies, the inter-departmental collaboration becomes effortless. This way, all the teams naturally start bouncing ideas off of each other and are motivated to share insights because they can see the direct impact on their day-to-day work. It’s fascinating. This approach has really helped in removing barriers between teams. Now, everyone works with the same numbers and facts, rather than each team having their own separate data.
Ira Prevalova, Growth Marketing Director, Adverity
Promote Continuous Learning
Dealing with big data projects is always a difficult process, where cooperation between departments can contribute to success or, conversely, delay it. To avoid the second scenario, I advise you to establish constant communication between teams, not just when needed. Organize joint meetings, after-hours visits, and training sessions that can strengthen team spirit and show all employees that they have a common goal.
One of the most common barriers to interdepartmental collaboration is the gap between the knowledge of different specialists. A technical employee may have difficulty understanding marketing strategies, and a PR manager may have difficulty setting up a program. Therefore, it is important to promote the idea of continuous learning, not only in a specific area, but for a general understanding of the main points. This will help eliminate the complexity of communication and facilitate the process of working with big data.
And do not forget to use common platforms that contain all the information about the project from each department. We work with Worksection, where each department can see the information they require at any time, without waiting for a response from a specific person and distracting them from their work. Your team should see the big picture and feel involved in the success of the project.
Finally, open and positive communication is the engine of the process. Big data projects are complex and difficult, and therefore require constant discussion and coordination. And this can only be achieved if your employees respect each other and share a common goal.
Evgen Kushnirchuk, Marketer, SEO Expert, and CEO, Hire Developers Biz
Related Articles
- Azure — a game changer for Big Data projects – TechBullion
- The Role of Big Data in Making Smarter Decisions – TechBullion
- Collaborative Strategies for Building Strong and Sustainable Business
Source: Collaboration in Big Data Projects: 16 Recommendations for Your Business